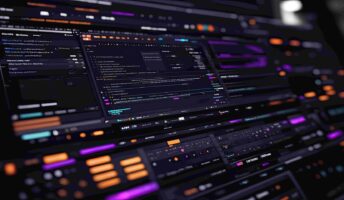
Don’t Let Your Staff Download These Browser Extensions
Today, browser extensions are ubiquitous. But as a recent malicious campaign shows, they're also a cybersecurity nightmare.
Today, browser extensions are ubiquitous. But as a recent malicious campaign shows, they're also a cybersecurity nightmare.
We track the latest AI errors and mistakes from 2024 and previous years, and catalog AI's worst failures to date.
Ingram Micro, which serves thousands of businesses around the world, is reportedly the latest victim of threat group SafePay.
Hackers are claiming responsibility for the breach that saw the data of up to six million customers exposed.
Equipment deductions and estate tax exemption are a few benefits, but ineligiblity for overtime remains.
Economic uncertainty and the rise of AI are creating some problems for college grads looking for employment.
Texas is leading the pack, with a huge percentage of businesses vowing to attract new truckers to the industry.
Over the last five years, cargo theft has risen substantially during the week of 4th of July.
The vote was 99-1, with significant bipartisan support for allowing states to regulate AI for the next ten years.
Anthropic gave its AI chatbot Claude a small store to run, and the results were... interesting.
Findings from a Thomson Reuters report reveals that businesses operating with AI strategies are already seeing ROI.
The digital services tax would have charged an estimated $2 billion to US tech giants when it went into effect on June 30th.
The DOT will be "reviewing the potential for unqualified individuals obtaining licenses," says the new announcement.
63% of companies said their difficulties with finding new drivers have remained the same or gotten worse in the last year.
Technology companies Kodiak and Vay revealed a partnership ahead of Kodiak's self-driving truck ambitions.
According to a new study, the main focus for tech leaders in 2025 is expanding the use of AI.
New AI tools include multicam, Weather Intelligence, and a TikTok-style coaching app that can reward safe driving.
Samsung already has a partnership in the works with Perplexity, potentially complicating an Apple buyout.
Microsoft seems to be getting in one last round of job cuts for its gaming division before its fiscal year ends on June 30th.
The 'low-key' launch didn't feature the Cybercab, but instead saw current Tesla models sporting a Robotaxi logo decal.
This isn't great news for the trucker shortage, which remains poised to cause some serious problems for the supply chain.